목록분류 전체보기 (185)
yuns
DFS BFS 동작 원리 stack queue 구현 방법 재귀 함수 이용 큐 자료구조 이용
가까운 노드부터 탐색하는 알고리즘 알고리즘 동작 방식 탐색 시작 노드를 큐에 삽입하고 방문 처리를 한다. 큐에서 노드를 꺼내 해당 노드의 인접 노드 중에서 방문하지 않은 노드를 모두 큐에 삽입하고 방문처리를 한다. 2번의 과정을 더 이상 수행할 수 없을 때까지 반복한다. from collections import deque def bfs(graph, start, visited): queue = deque([start]) visited[start] = True #queue가 빌 때까지 반복함을 의미 while queue: v = queue.popleft() print(v, end=' ') for i in graph[v]: if not visited[i]: queue.append(i) visited[i] =..
그래프 구조 - 노드(node, vertex)와 간선(edge)로 이루어짐 인접행렬 방식: 2차원 배열로 그래프의 연결 관계를 표현하는 방식 INF = 99999999 graph = [ [0, 7, 5], [7, 0, INF], [5, INF, 0] ] 인접리스트 방식: 연결리스트로 그래프의 연결 관계를 표현하는 방식 graph = [[] for _ in range(3)] #node k와 edge 크기 n으로 연결된 node d에 대하여: graph[k].append((d, n)) #노드 0에 연결된 노드들에 대한 정보 graph[0].append((1, 7)) graph[0].append((2, 5)) #노드 1에 연결된 노드들의 정보 graph[1].append((0, 7)) #노드 2에 연결된 노드..
탐색: 많은 양의 데이터 중에서 원하는 데이터를 찾는 과정 자료구조: 데이터를 표현하고 관리하고 처리하기 위한 구조 스택(Stack): FILO, LIFO 구조 append(), pop()메소드 사용 st = [] st.append(2) st.append(3) st.pop() 큐(Queue): FIFO구조 from collections import deque queue = deque() queue.append(5) quque.append(2) quque.popleft() #for printing queue.reverse() 재귀함수(Recursive Function): 자기 자신을 다시 호출하는 함수 함수로 정의하고 호출하는데 너무 많이 호출하게 될 경우 RecursionError 발생 재귀함수의 종료조..
Four Limitations Computationally inefficient to update the hidden states of nodes iteratively to get the fixed point. uses the same parameters in the iteration while most opoular neural networks use different parameters in different layers, which serves as a hierarchical feature extraction method some informative edges(Knowledge Graph) cannot be effectively modeled in GNN. if T is pretty large, ..
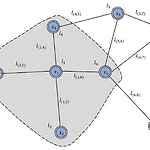
$$h_v = f(x_v, x_{co[v]}, h_{ne[v]}, x_{ne[v]}) $$ $$o_v = g(h_v, x_v)$$ Functions $f$: local transition function which is shared among all nodes $g$ : local output function Symbols x: the input feature h: hidden state $co[v]$: the set of edges connected to node $v$ $ne[v]$: the set of neighbors of node $v$ $x_v$: the features of $v$ $x_{co[v]}$: the features of its edges $h_{ne[v]}$: the states..
ieeexplore.ieee.org/abstract/document/4700287/ [IEEE 09] The Graph Neural Network Model 작성 기준 1624회 인용 The Graph Neural Network Model - IEEE Journals & Magazine ieeexplore.ieee.org Node는 각각의 feature들과 연결되어 있는 node들로 표현된다. GNN의 target to learn a state embedding $h_v \in \mathbb{R}^s$ encodes the information of the neighborhood 이웃들의 정보를 encoding $h_v$는 output인 $o_v$을 만들어내기 위해 사용됨 논문에서 "Undirected ..
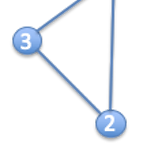
참조: (번역) medium.com/watcha/gnn-%EC%86%8C%EA%B0%9C-%EA%B8%B0%EC%B4%88%EB%B6%80%ED%84%B0-%EB%85%BC%EB%AC%B8%EA%B9%8C%EC%A7%80-96567b783479 GNN 소개 — 기초부터 논문까지 이 글은 Shanon Hong의 An Introduction to Graph Neural Network(GNN) For Analysing Structured Data를 저자에게 허락받고 번역, 각색한 글이다. medium.com 참조: (원본) towardsdatascience.com/an-introduction-to-graph-neural-network-gnn-for-analysing-structured-data-afce79f4..